The global community’s expectations for corporations have changed as climate-related catastrophes continue to strike with increasing devastation. In 2020, Bloomberg investigated board members across 20 European and U.S. organizations and found that “the greening of global finance had not yet reached the boardroom.” As the world reels from a turbulent geopolitical landscape, the vulnerabilities and volatility of the energy market, and a heightened urgency for energy independence, board directors worldwide are beginning to discuss ESG and tactics and actions for managing decarbonization and integrating climate change into their oversight responsibilities.
It is international scientific consensus that, to prevent continued climate damage, global net human-caused emissions of carbon dioxide (CO2) will have to fall by 45 percent from 2010 levels by 2030, reaching net zero around 2050.

The role of the corporate board is changing. The world’s most prominent corporations are clamoring to demonstrate how seriously they are taking climate change, with an increasing number pledging to eliminate their carbon footprints in the decades ahead. The topic is now a firm fixture on board agendas. To rebuild corporate structures to conduct the policy, actions, and affairs necessary for the new world, all eyes are on the expansion and widespread adoption of renewable energy.
In 2020, worldwide renewable capacity increased by 45 percent or about 280 GW – the highest year-on-year increase since 1999. In 2021, worldwide renewables accounted for 90 percent of new capacity expansion. The United States is on track to generate 24 percent of its energy from renewables by the end of this year, owing to rapid growth in solar and wind capacity. In the next decade, the U.S. will build roughly 100 GW of wind and solar capacity annually to hit its longer-term climate targets.While these are great strides, more must be done to meet the aggressive 2030 and 2050 goals. Renewable energy operators will require maximized operational efficiency and production.
In this era of widespread digital transformation and the IIoT, renewable assets generate vast amounts of data, but providers are challenged with transforming their raw data into actionable insights that drive operational excellence. Additionally, energy operators are increasingly diversifying the kinds of assets in their fleets, often managing a mix of wind, solar, and battery storage assets simultaneously under the same project. While there are some synergies between these different asset types, optimizing the performance of a mixed fleet is challenging.
Artificial Intelligence (AI) is already doing some heavy lifting in the day-to-day operations of wind, solar, and battery storage assets. By fully leveraging AI, operators can:
Improve operational efficiency by democratizing access to data to all that can benefit from it.
Maximize wind-energy production by quickly detecting and correcting under performance due to yaw misalignment, control system issues, and other factors.
Minimize unplanned maintenance costs by predicting component failures months in advance, and in some cases preventing costly failures from occurring.
Increase solar production with enhanced O&M practices to detect and quantify panel soiling, identify stuck trackers, and surface combiner box issues.
Production Forecasting
The probabilistic and variability of wind energy distinguishes it from other renewable resources. For farms with large-scale capacity, a wind-speed variation of 1 m/s can influence power generation significantly, which makes forecasting critical to help guarantee power system reliability and to help balance supply and demand.
An industry-specific AI model can accurately forecast variable wind-energy production to help manage supply and demand fluctuations. A single cloud-based SaaS solution can manage multiple asset types — wind, solar, and battery energy storage — and provide a comprehensive solution suite for holistic management that supports ISPs, IPPs, and utilities. The result is increased energy production, reductions in maintenance costs, and improved operational efficiency. Wind power becomes more profitable, which creates the catalyst for being more widely used in power systems worldwide.

Identifying Wind-Turbine Yaw Misalignment
With the increased and rapid development of large-scale wind turbines, yaw misalignment has become a large-scale issue. Static yaw misalignment degrades turbine power production and can create blade fatigue, and because it’s invisible to the turbine controller, specialized methods are required to identify and address it.
The legacy methods for identifying yaw misalignment were light detection and ranging (Lidar) measurement or physics-based methods applied to turbine SCADA data. Both techniques have significant disadvantages compared to AI. With Lidar, every turbine requires its own sensor, which makes it cost prohibitive, and physics-based methods require data over an extended period, typically a year, when yaw misalignment is present, which makes it time prohibitive.
A machine learning-based solution can act as an extension of the physics-based method by using only two months of historical data to train the machine learning model to detect a yaw misalignment of five degrees or more with 96 percent accuracy. In the event of a misalignment (positive or negative), a controller is automatically notified of the magnitude and direction of yaw so the issue can be rectified expeditiously. An AI cloud computing platform operates 24/7 with no input required from controllers. Because there are fewer tower climbs, safety risks or additional costs are minimized, and turbines operate optimally.
As an example of efficacy, an operator updated the turbine yaw offset remotely, resulting in as much as 2 percent additional Annual Energy Production (AEP) — an annual revenue increase of $45,000/turbine for the project.
Predicting Pitch Bearing Failures
Pitch bearing failure is a significant issue for the wind industry. Pitch bearings connect the rotor hub to the blades to facilitate the required oscillation, which affects the lifting force of the horizontal turbine to rotate. They experience static and dynamic forces throughout a turbine’s lifetime, presenting varying modes of failure. The costs associated with repair or replacement are significant, especially if the turbine is offshore. In an extreme case, a pitch bearing failure can accelerate or advance the loss of a blade, which comes at quite a cost. As of May 2022, a single wind-turbine blade costs an average of $2.6 million to $4 million.
The traditional method of diagnosing pitch bearing health is by taking samples of the lubricant from the bearings and analyzing them. The quantity and size of the wear particles in the lubricant provide some insights into the health of the bearing, but obtaining samples is both time-consuming and expensive. The inoperable turbine must be taken offline, and technicians must enter the hub to pull samples.
Predicting bearing failures in advance can significantly reduce unscheduled operations and maintenance costs. AI uses data from existing sensors on the turbine to detect pitch bearing issues remotely. Signals can include commanded pitch position, actual pitch position, pitch motor current and temperature (for electric pitch systems), pitch system pressures (for hydraulic pitch systems), turbine fault history, and other relevant signals.
With an AI platform explicitly built for renewables, the pitch bearing health algorithms run on a cloud computing platform 24 hours a day, with no input required from analysts or controllers. The algorithms use only existing signals from the turbines, so no additional hardware is required. The data required for the algorithms are continually sent to the platform without taking the system offline or physically climbing the turbine. When a faulty pitch bearing is detected, it automatically notifies controllers so the damage can be confirmed.
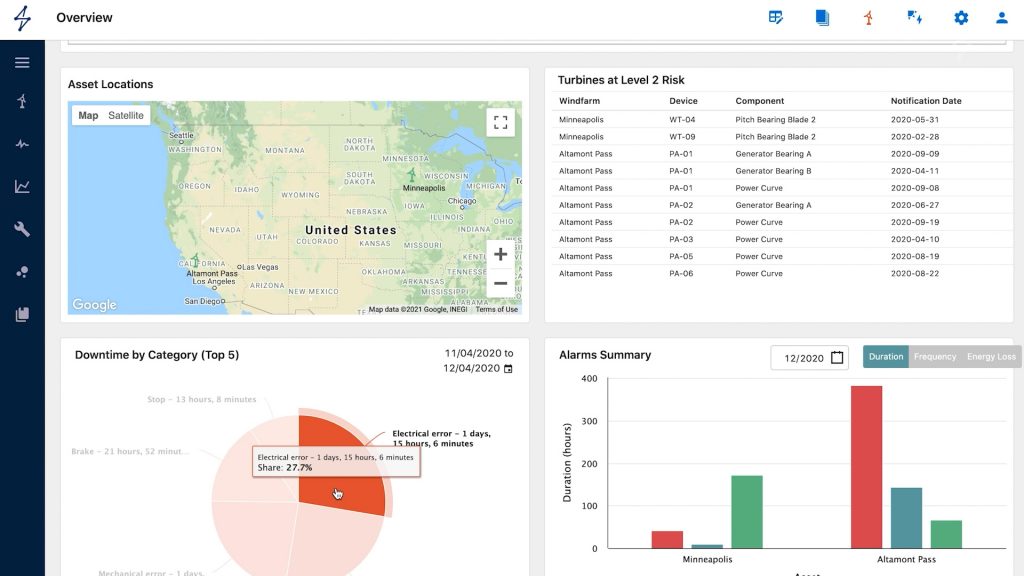
AI can predict failures with more than 90 percent accuracy up to six months in advance, which gives operators a long enough lead time to put replacement bearings on order, thereby minimizing downtime and repair expenses.
Boost Profitability by Maximizing Energy Production
Another challenge for wind-turbine owners and operators is understanding the causes of energy loss and the subsequent revenue shortfalls. Wind farms can range in size from only a handful of turbines to more than 100. They also vary in terrain from flat and low-slope areas to very complex, highly turbulent areas.
With the repowering of older sites, many wind farms have mixed turbine types, making understanding actual energy losses and their causes far more problematic. It applies to both self-performing wind farms and those under full-wrap supply agreements.
The typical method for calculating lost turbine energy is to use the nearest neighbor to calculate the energy loss during downtime. This method provides accurate results under a very narrow set of cases, such as a wind farm with flat terrain and significant distance between turbines. For more typical wind farms with AAI effects, complex terrain, and mixed turbines, the calculated energy losses using this method can be off by as much as 10 percent. It adversely affects the calculation of Availability Damages or Availability Bonuses and the operator’s bottom line.
Accurate calculations of wind-power energy losses are critical for owners with or without an OEM warranty. Because wind farms are data rich, physics-informed machine learning approaches can provide a more accurate framework to calculate losses.
For example, an owner-operator of more than 1 GW of wind assets with wind farms ranging from just a few MW to more than 200 MW has a fleet of multiple OEMs. The challenge was to provide a unified view of all their assets and reconcile energy losses provided by all the OEMs, which only provided energy loss results for downtime related to turbine unavailability. There was a complete lack of visibility into revenue lost due to Balance of Plant (BOP) processes or contractually excluded downtime. Traditional energy loss calculations can result in significant errors. Given appropriate input variables, including wind direction, velocity, and power for each turbine, AI can predict the power of a wind farm accounting for the wake losses, determine whether a correction is required, and improve the accuracy of loss calculations.
In another situation, when a site had a wind-farm section down for more than a month because of an electrical outage, AI calculated energy loss due to BOP issues such as a feeder failure or substation outage. The OEM did not report the energy loss because it was outside their scope. There was no SCADA data available for the timeframe for the affected turbines. The AI model calculated the energy loss for the affected section of the project. The energy loss was significantly higher than the owner’s calculation method, and the energy-based BOP availability was reduced by more than 8 percent. In addition to an accurate accounting of the energy loss, it guided the owner’s decisions for spare parts inventory planning.
Accurately calculating losses due to grid curtailment is also challenging for operators. Project economics related to curtailment is often based on an average wind speed across a particular site using a single power curve. For a wind farm with many mixed turbines, using a single power curve calculation methodology is destined to be flawed.

Using a data-driven method allows owners and operators to accurately calculate the energy loss from curtailment and get accurate curtailment compensation from the offtaker. AI can help operators receive the true value from their O&M contracts and help owners understand where energy is being lost so that they can focus their efforts on corrective actions with the highest ROI.
PV Soiling and Panel Misalignment
For renewable energy providers whose mixed assets include photovoltaics (PV), environmental conditions often affect photovoltaic performance. Panels accumulate dust, pollen, dirt, algae, snow, bird droppings, and other debris, which reduces the ability to turn solar energy into electric power. Uneven soil patches can also affect some PV module cells, which disrupts the flow of current throughout the entire module. It creates hot spots that eventually damage sensitive components, shortening panel lifespan. According to Solar Energy Power Association, dirty solar panels can lose 20 percent of their energy output.
The National Renewable Energy Laboratory (NREL) puts that figure even higher, at 25 percent. In arid regions like the Middle East, energy output reductions due to soiling can reach 50 percent per year, creating multi-billion dollar revenue losses.
Soiling also creates higher operating costs with constant equipment monitoring, cleaning, and replacing damaged assets. A renewable-specific AI-powered asset management platform can ingest the available SCADA data while contextualizing current and future weather conditions, project capacity, power purchase agreements, and curtail protocols. The technology can help solar power operators quantify the economic value of lost production caused by panel soiling and identify ways to address the issue most efficiently by interpreting weather forecast data.
Operators can determine when a precipitation event of sufficient magnitude to clean the panels will occur. All the data sources are combined with the actual cost of cleaning the panels, and an optimization process provides the project operator with the ideal time to perform panel cleaning for maximum project profitability and eliminate the need for soiling stations.
Conclusion: Powering a Safer Future
Society’s expectations are finally beginning to influence the way companies operate. The impetus for corporations to switch to renewable energy for manufacturing, industry, and service is steeped by the aspiration of a future defined by clean energy. It diversifies energy sources to hedge against fluctuating fossil fuel prices and mitigates inclement weather service disruptions. It also positively touches the bottom line, reduces corporate greenhouse gas emissions, and strengthens stakeholder relationships and brand differentiation.
Renewable energy has experienced an exponential growth trajectory, making it the fastest-growing energy source globally. The industry is also realizing increased complexities of hybrid power generation sources, increasing pressure on profit margins, and shifting workforce demographics. In the face of these challenges, renewable energy must keep pace with the demand to facilitate the transition from fossil fuels while making the most of their capital and human investments.
Artificial intelligence is the catalyst to full-feature performance optimization that owners and operators need to ensure continuously profitable operations, enabling them to deliver on Power Purchase Agreement (PPA) commitments while ensuring worker safety and the achievement of sustainability goals. Artificial intelligence is uniquely positioned to enable that vision as the world races to unwind a century of fossil-fuel-powered energy production.