Wind-energy research plays a vital role in the possibility of the success story of wind energy as one of the most promising sustainable energy sources. This continuous process has been achieved from the era of small wind turbines to the current multi-MW standard and beyond.
1 Introduction
Wind energy has become an essential part of human life worldwide and has undergone massive development in different areas in recent decades. This is driven by the constant depletion of fossil fuels, fear of the energy crisis, price volatility, and people’s awareness of cleaner and greener sources of energy. Wind energy is one of the most promising and mature sources of sustainable energy in terms of technology and market readiness. In fact, the growth of wind energy is considered as the fastest among other renewable energy sources. The success story of wind energy is tightly coupled with the long history in its research and development in many aspects. This includes the continuous effort spent on improving the reliability of wind turbine designs and site assessments.
The rapid growth of wind energy raises new challenges, which need to be addressed accordingly. One of the major concerns in wind-energy development is the space availability and the increased demand for wind power. The fear of an energy crisis makes it worse because a significant proportion of the current energy supply is still reliant on fossil fuels.
In contrast, renewable energy sources, such as wind energy, still cannot replace the fossil fuel dependency completely. The design of wind turbines, for instance, has established a clear direction to increase the power of each turbine up to 10 to 15 MW, and may be much higher in the future. This surely poses a completely different challenge compared to the wind-turbine technology in the past, e.g., a large wind turbine has much, much longer, more slender, and flexible blades. On the other hand, centralized power stations adopting these gigantic machines may not be suitable for remote areas with limited grid connectivity. This is where a decentralized energy concept using small wind turbines has found its place and is considered a viable solution.
The discussions below provide an overview of the trend of wind-energy developments that challenge the current research orientation. This article is aimed at discussing the direction of the current wind-energy research and its importance to meet future expectations. This article compiles and provides the key findings of several recent studies, and it is focused on the technical aspects of wind-energy modeling. Most studies were collected from articles published in Energies within the last two to three years and several selected studies from various publications. Two main topics will be discussed in this article: (1) in the area of wind-turbine design and computations and (2) in the usage of data-driven approaches.
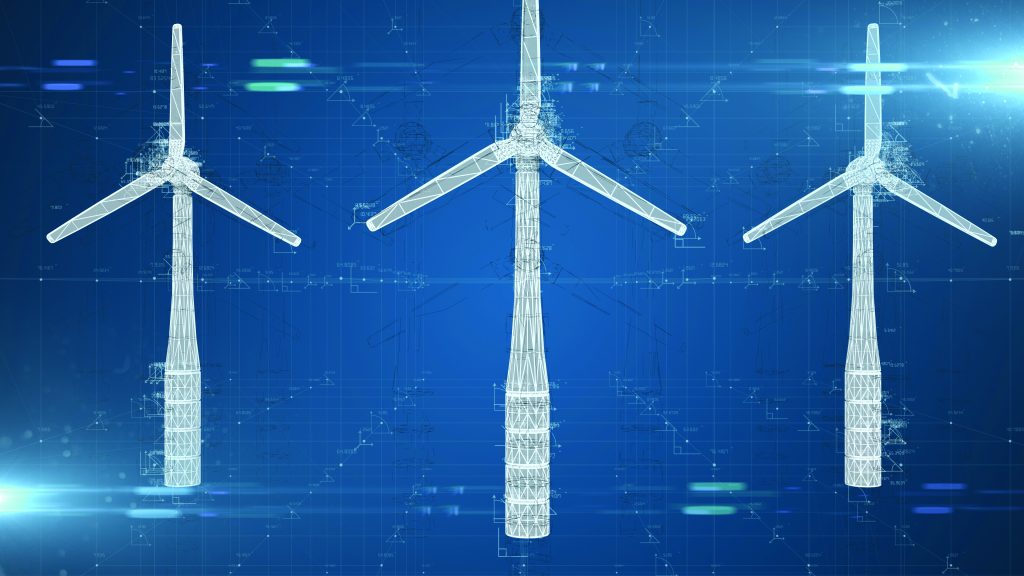
2 Challenges in Wind-Turbine Design and Prediction Tools
Wind-turbine design is one of the most important aspects in ensuring the generated power and the lifetime of the turbine meet the expected demand. As a part of this process, the resulting loads must be measured or estimated correctly. It is agreed that the reliability of wind-turbine load assessments depends strongly upon the accuracy of wind-turbine prediction tools themselves. In practice, load-case analyses are performed to evaluate wind-turbine loads and performance under various conditions, including turbulent wind, controller fault, extreme conditions, etc.
Progress has been made in ensuring the accuracy of common prediction tools with various fidelity levels for wind-turbine analysis. Michna et al. [1] provided a comprehensive assessment of different computational fluid dynamics (CFD) codes in predicting the transition process of a wind-turbine airfoil. The studies were compared with experimental data and an integral boundary layer code. It was confirmed the calculated aerodynamic force coefficients from different CFD codes are consistent despite the different mesh distributions used. Tagliafierro et al. [2] performed a study that was aimed at establishing and validating an initial setup for a tension-leg platform for floating offshore wind turbines (FOWTs) with a meshless code. The study concluded that meshless CFD models can provide reasonable accuracy in simulating turbine operating conditions. It was suggested to further explore the characteristics for different scenarios such as for semi-submersible platforms.
Li and Yang [3] compared the degree of flow dynamics in wind-turbine wake obtained from actuator disk and actuator surface computations. The studies revealed the agreement of both methods depends on the inflow conditions. Because numerical studies were usually conducted independently from one author to the other authors with various codes, it is often difficult to derive the exact conclusion when the same turbine model is evaluated at a later time. Collective comparative studies such as those reported by the International Energy Agency (IEA) can be useful in assessing the coherence of codes with different fidelity levels to model turbine physics [4,5].
Further investigations were performed for offshore wind turbines’ specific cases. Cottura et al. [6] modeled an offshore floating wind turbine and examined the behavior resulting from a wide spectrum of sea and wind states typical of the Mediterranean Sea. The turbine levelized cost of energy (LCOE) was further evaluated. The studies indicated there is a tight relationship between the environmental conditions and the cost of energy. Liu and Yu [7] also performed a study that attempted to model the complex dynamics of SPAR-type FOWTs under wave group scenarios. It was concluded the natural frequency responses increase with corresponding frequency wave loads, suggesting that the amplified motions may endanger the system in operation.
The usage of numerical tools is also proven to be helpful for improving the geometry of a wind turbine. Khlaifat et al. [8] demonstrated the design of a wind turbine could be optimized based on the local wind conditions, highlighting the design of wind turbine might be adjusted depending on the site location for obtaining the best performance. Further optimization was also carried out by adding passive flow controls, such as Gurney flaps, e.g., by Chakroun and Bangga [9], Gupta et al. [10], and Wiśniewski et al. [11].
Numerical tools also may be used to improve the turbine performance by airfoil reshaping, e.g., by Bangga et al. [12] and Kim et al. [13]. Lee and Shin [14] adopted the blade-element momentum (BEM) theory as a tool to compute the wind-turbine loads for wind-turbine design optimization process. BEM has been proven to be suitable even for a very large wind turbine having a 10-MW rated power [15,16]. Rogowski et al. [17] also confirmed numerical approaches can be used to assist the selection of the most optimal airfoil shape for a particular wind-turbine type.
To fully understand the characteristics of wind-turbine loads, a comprehensive inclusion of aeroelastic effects in CFD will be necessary. Lipian et al. [18] provided an example of fluid–structure interaction (FSI) studies for a small wind turbine. Although it was already expected, the aeroelastic effects will have little influence on small rotors, the studies revealed FSI is still beneficial for the strength analysis. Tian et al. [19] conducted an FSI study for a 1.5-MW wind turbine rotor having a diameter of about 84 meters during strong winds. The main aim of the study was to explore the stress distribution, as well as the damage to the turbine blades. By combining the results of the numerical simulation with the experimental data, the study provided the location of typical failure areas. Using an FSI approach, Santo et al. [20] managed to evaluate the characteristics of a large turbine having a diameter of 100 meters under the influence of wind gusts. The study showed an initial decrease in the rotor blade loads and displacement is a consequence of the negative velocity augmentation at the gust border. The structural inertia causes a tip movement delay when the gust positive core acts on the blade. Although a high peak is reached by the axial force on the blade, the occurrence of flow separation over the span prevents the blade from reaching extreme deflections. Several other studies also highlighted three-dimensional separated flow for a rotating blade could be influenced by various physical effects such as the Coriolis and centrifugal forces, as well as unsteady effects [21–24].
3 USING Data in Wind-Turbine Assessment
Data-driven approaches have gained their momentum in recent years in various aspects including wind-energy sector. This area also received a huge interest in the wind-energy research community as documented in numerous studies [25-31]. These studies covered a wide area of interests, such as wind-turbine modeling, time-series forecasting, and health monitoring.
Very recently, Geibel and Bangga [25] compared various data-driven algorithms to reconstruct the wind-turbine wake flow field and time-series under realistic inflow conditions. The time-series prediction was based on the bi-directional long short-term memory (Bi-LSTM) approach and was verified using data from high-fidelity CFD simulations.
The obtained results showed data-driven approaches for wind-turbine wake prediction could offer an alternative to conventional prediction approaches. Delgado and Fahim [27] also suggested the LSTM-based method is suitable for short-term time-series prediction by using a database from a supervisory control and data acquisition (SCADA) system.
Donadio et al. [26] further attempted to combine a numerical weather prediction with a machine learning approach for wind-assessment purposes. This study also highlighted that machine learning is helpful and suitable for such prediction tasks. Data-driven approaches have also been used to model the turbine performance and health condition. Lin and Liu [30] applied a deep-learning approach to study the major driven force on the mooring line tension for floating offshore wind turbines. The study considered a number of conditions from cut-in to cut-out wind speeds. It was concluded the mooring loading is mainly determined by the surge motion. In contrast, this study demonstrated the blade and the tower elasticity have fewer consequences.
Rushdi et al. [31] attempted to employ experimental data to train machine learning regression models of an airborne wind-energy system. In agreement with the above observations, this study also suggested the great promise of machine learning in wind-turbine prediction tasks. Santolamazza et al. [28] proposed a methodology based on machine-learning techniques with the data obtained from a SCADA system. The main objective was to characterize the behavior of some of the main components of wind turbines (such as gearbox and generator) and to predict operating anomalies. The adopted method was tested on wind turbines in Italy to evaluate its suitability, and it was demonstrated to successfully provide an aid for the maintenance of a wind farm. In a similar field, Kang et al. [29] adopted a support vector machine (SVM) to achieve an optimized economy and availability by an opportunistic maintenance policy. The study indicated the data-driven condition-based maintenance policy is helpful in reducing the maintenance expenditure of offshore wind turbines.
Mentioned studies demonstrated data-driven approaches are beneficial in wind-energy analysis in various areas. It is agreed the main challenges for most models are the source of the data and the real practical implementations.
Funding
This research received no external funding.
Data Availability Statement
Data can be made available by contacting the corresponding author.
Conflicts of Interest
The author declares no conflicts of interest.
About the author
Dr. Galih Bangga has collected years of experience in the field of wind-turbine aerodynamics as a senior engineer at DNV in the U.K. and as a scientist at the University of Stuttgart in Germany. Copyright: © 2022 by the author. Licensee MDPI, Basel, Switzerland. This article (https://www.mdpi.com/1996-1073/15/18/6527/pdf) is an open access article distributed under the terms and conditions of the Creative Commons Attribution (CC BY) license (https://creativecommons.org/licenses/by/4.0/). It has been edited to conform to the style of Wind Systems magazine.
References
- Michna, J.; Rogowski, K.; Bangga, G.; Hansen, M.O. Accuracy of the gamma re-theta transition model for simulating the DU-91-W2-250 airfoil at high Reynolds numbers. Energies 2021, 14, 8224.
- Tagliafierro, B.; Karimirad, M.; Martínez-Estévez, I.; Domínguez, J.M.; Viccione, G.; Crespo, A.J. Numerical Assessment of a Tension-Leg Platform Wind Turbine in Intermediate Water Using the Smoothed Particle Hydrodynamics Method. Energies 2022, 15, 3993.
- Li, Z.; Yang, X. Evaluation of actuator disk model relative to actuator surface model for predicting utility-scale wind turbine wakes. Energies 2020, 13, 3574.
- Schepers, J.; Boorsma, K.; Madsen, H.A.; Pirrung, G.; Bangga, G.; Guma, G.; Lutz, T.; Potentier, T.; Braud, C.; Guilmineau, E.; et al. IEA Wind TCP Task 29, Phase IV: Detailed Aerodynamics of Wind Turbines; International Energy Agency: Paris, France, 2021.
- Boorsma, K.; Schepers, G.; Aagard Madsen, H.; Pirrung, G.; Sørensen, N.; Bangga, G.; Imiela, M.; Grinderslev, C.; Meyer Forsting, A.; Shen,W.Z.; et al. Progress in validation of rotor aerodynamic codes using field data. Wind Energy Sci. Discuss. 2022, 1–31.
- Cottura, L.; Caradonna, R.; Ghigo, A.; Novo, R.; Bracco, G.; Mattiazzo, G. Dynamic modeling of an offshore floating wind turbine for application in the Mediterranean Sea. Energies 2021, 14, 248.
- Liu, B.; Yu, J. Dynamic Response of SPAR-Type Floating Offshore Wind Turbine under Wave Group Scenarios. Energies 2022, 15, 4870.
- Khlaifat, N.; Altaee, A.; Zhou, J.; Huang, Y.; Braytee, A. Optimization of a small wind turbine for a rural area: A case study of Deniliquin, New South Wales, Australia. Energies 2020, 13, 2292.
- Chakroun, Y.; Bangga, G. Aerodynamic characteristics of airfoil and vertical axis wind turbine employed with Gurney flaps. Sustainability 2021, 13, 4284.
- Gupta, A.; Rotea, M.A.; Chetan, M.; Sakib, M.S.; Griffith, D.T. A methodology for robust load reduction in wind turbine blades using flow control devices. Energies 2021, 14, 3500.
- Wiśniewski, P.; Balduzzi, F.; Buliński, Z.; Bianchini, A. Numerical analysis on the effectiveness of gurney flaps as power augmentation devices for airfoils subject to a continuous variation of the angle of attack by use of full and surrogate models. Energies 2020, 13, 1877.
- Herrmann, J.; Bangga, G. Multi-objective optimization of a thick blade root airfoil to improve the energy production of large wind turbines. J. Renew. Sustain. Energy 2019, 11, 043304.
- Kim, Y.; Bangga, G.; Delgado, A. Investigations of HAWT Airfoil Shape Characteristics and 3D Rotational Augmentation Sensitivity Toward the Aerodynamic Performance Improvement. Sustainability 2020, 12, 7597.
- Lee, S.L.; Shin, S. Wind turbine blade optimal design considering multi-parameters and response surface method. Energies 2020, 13, 1639.
- Bangga, G. Comparison of blade element method and CFD simulations of a 10 MW wind turbine. Fluids 2018, 3, 73.
- Schepers, J.; Boorsma, K.; Sørensen, N.; Sieros, G.; Rahimi, H.; Heisselmann, H.; Jost, E.; Lutz, T.; Maeder, T.; Gonzalez, A.; et al. Final results from the EU project AVATAR: Aerodynamic modelling of 10 MW wind turbines. In Journal of Physics: Conference Series; IOP Publishing: Bristol, UK, 2018; Volume 1037, p. 022013.
- Rogowski, K.; Hansen, M.O.L.; Bangga, G. Performance analysis of a H-Darrieus wind turbine for a series of 4-digit NACA airfoils. Energies 2020, 13, 3196.
- Lipian, M.; Czapski, P.; Obidowski, D. Fluid–structure interaction numerical analysis of a small, urban wind turbine blade. Energies 2020, 13, 1832.
- Tian, K.; Song, L.; Chen, Y.; Jiao, X.; Feng, R.; Tian, R. Stress Coupling Analysis and Failure Damage Evaluation of Wind Turbine Blades during StrongWinds. Energies 2022, 15, 1339.
- Santo, G.; Peeters, M.; Van Paepegem,W.; Degroote, J. Fluid–structure interaction simulations of a wind gust impacting on the blades of a large horizontal axis wind turbine. Energies 2020, 13, 509.
- Bangga, G.; Lutz, T.; Jost, E.; Krämer, E. CFD studies on rotational augmentation at the inboard sections of a 10 MW wind turbine rotor. J. Renew. Sustain. Energy 2017, 9, 023304.
- Brownstein, I.D.; Wei, N.J.; Dabiri, J.O. Aerodynamically interacting vertical-axis wind turbines: Performance enhancement and three-dimensional flow. Energies 2019, 12, 2724.
- Bangga, G.; Hutani, S.; Heramarwan, H. The Effects of Airfoil Thickness on Dynamic Stall Characteristics of High-Solidity Vertical AxisWind Turbines. Adv. Theory Simul. 2021, 4, 2000204.
- Robinson, M.C.; Hand, M.; Simms, D.; Schreck, S. Horizontal Axis Wind Turbine Aerodynamics: Three-Dimensional, Unsteady, and Separated Flow Influences; Technical Report; National Renewable Energy Lab. (NREL): Golden, CO, USA, 1999.
- Geibel, M.; Bangga, G. Data Reduction and Reconstruction of Wind Turbine Wake Employing Data Driven Approaches. Energies 2022, 15, 3773.
- Donadio, L.; Fang, J.; Porté-Agel, F. Numerical weather prediction and artificial neural network coupling for wind energy forecast. Energies 2021, 14, 338.
- Delgado, I.; Fahim, M. Wind turbine data analysis and LSTM-based prediction in SCADA system. Energies 2020, 14, 125.
- Santolamazza, A.; Dadi, D.; Introna, V. A data-mining approach for wind turbine fault detection based on SCADA data analysis using artificial neural networks. Energies 2021, 14, 1845.
- Kang, J.; Wang, Z.; Guedes Soares, C. Condition-based maintenance for offshore wind turbines based on support vector machine. Energies 2020, 13, 3518.
- Lin, Z.; Liu, X. Assessment of wind turbine aero-hydro-servo-elastic modelling on the effects of mooring line tension via deep learning. Energies 2020, 13, 2264.
- Rushdi, M.A.; Rushdi, A.A.; Dief, T.N.; Halawa, A.M.; Yoshida, S.; Schmehl, R. Power prediction of airborne wind energy systems using multivariate machine learning. Energies 2020, 13, 2367.