Wind power is emerging as a sustainable source of electricity. As we know, finding solutions for improving the reliability and durability of gearboxes is a long-standing objective for the lubricant industry.
Wind farms tend to be in remote locations, whether on land or offshore. In a Tribology and Lubrication Technology article [1], a study that complemented a 2015 report on offshore wind energy by the U.S. Department of Energy (DOE) was discussed. The authors of the follow-up study indicated that the DOE should have factored in the availability of the rare earth metal neodymium because it is needed for direct drive systems, which are preferred for use offshore. As part of this work, the authors divided the U.S. into five distinct regions and developed what-if scenarios to predict the potential use of neodymium for the next 30 years in each region.
Besides the challenges just discussed, optimizing the electricity generated is difficult to do because of regional differences in the magnitude of the wind power. Predicting the wind speed in any specific location even 24 hours ahead is difficult to forecast.
“A power company, reliant on wind power, must plan on a daily basis how much energy will be provided,” said Guido Cervone, professor of geography, meteorology, and atmospheric pressure at Penn State. “The answer will prompt the power company to determine how much energy they may need to buy or sell on any given day. Costs will rise if the power company must continue to buy electricity from other sources if they cannot secure the anticipated amount from wind power.”
There are several methods available to predict wind speed that have been organized into the following categories: numerical weather prediction models, statistical models, artificial neural network models, and hybrid models. Some of these models are better at short-term forecasting (30 minutes to six hours ahead), while others contribute better to long-term forecasting (one day to one week ahead).
“Each of these models has inaccuracies that are analogous to the cone of uncertainty and spaghetti models seen in weather forecasting,” Cervone said. “One crucial factor for the operational use of renewable energy is to estimate how well models are able to predict future outcomes. This predictability parameter now proves to be useful in using past wind data to predict the future.”
Cervone, Dr. L. Delle Monache of Center of Western Water Extremes, Scripps Institution of Oceanography at the University of California-San Diego, and his colleagues used predictability in a new technique to prepare a probability curve for wind speed across the U.S.
The Analog Ensemble Approach
The researchers used a model known as the Analog Ensemble approach to determine wind speeds at specific locations.
“The Analog Ensemble is the brainchild of Dr. Delle Monache, and it was first developed at the U.S. National Center for Atmospheric Research,” Cervone said. “It generates probabilistic forecasts for future outcomes by analyzing past performance of forecasts. A two-dimensional grid determines the probability distribution of wind speeds using variables such as temperature and pressure over space and time.”
The forecasting was done for wind that is 80 meters above sea level.
“We used this altitude because it is the height of the hub of the turbine, which is the component connecting the blades to the main shaft,” Cervone said.
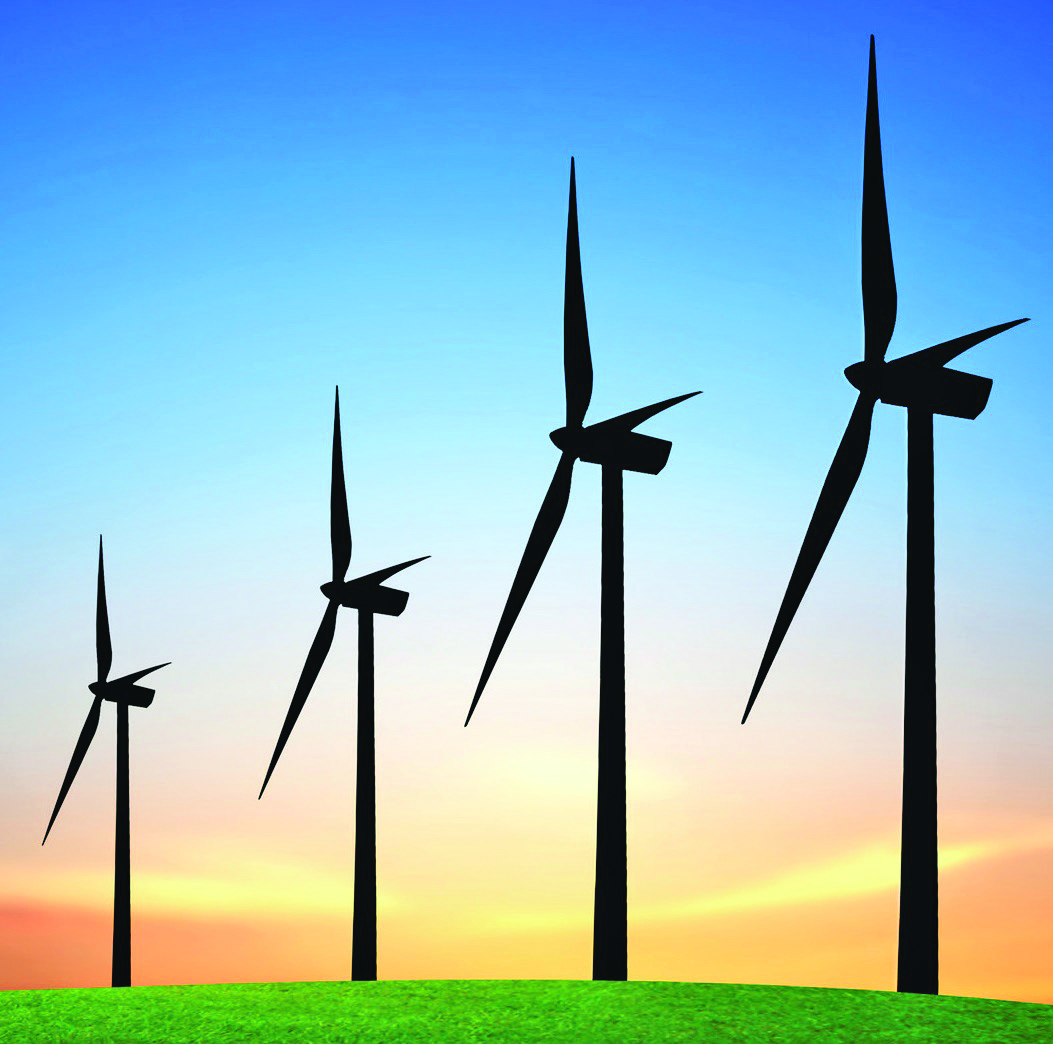
For a specific location, data from the past is used that matches as closely as possible the location’s time of year in order to predict the future.
“In our efforts to predict wind speed 24 hours in advance, the model selected specific dates from the past (such as January 1, 2016, March 15, 2016, and November 10, 2016) that have the same weather as is predicted at the time of forecasting,” Cervone said. “The database of past weather we used extended from January 15, 2015, through January 14, 2017. We used six-hour intervals in using the model to predict wind speeds.”
The challenge for the researchers is dealing with extreme weather events.
“Weather events that do not necessarily occur routinely at a specific location are more difficult to factor into the Analog Ensemble model,” Cervone said.
Extreme weather is more likely to be found in regions where the average wind speed is higher.
“An operator can use this model to place a wind farm in a specific region with a fair chance that the electricity generated will meet the specific demands of a utility,” Cervone said. “Some measure of uncertainty will always be present, leading to the fact that locating a wind farm anywhere always has a certain degree of risk.”
The researchers are determining how to use this model to account more accurately for extreme and rare weather events.
“We also are evaluating every grid in the U.S. to determine how to better improve the accuracy of the model,” Cervone said.

Recognition exists that wind farms operate optimally when the wind blows at a low rate of speed and is fairly consistent. Cervone is fairly confident that the model produced in this study will be useful for assisting the wind-turbine industry with situating turbines in locations that can generate electricity at an optimum rate to meet current and future demand.
“Our model will not tell operators where to place a wind farm but, rather, assist them with understanding the probability of having the proper wind conditions to produce electricity successfully in any specific location,” he said.
From the lubricant standpoint, wind turbines operating under fairly consistent conditions will lead to extending the durability of gear oils. Additional information on this research can be found in a recent article [2] or by contacting Cervone at cervone@psu.edu.
REFERENCES
- Canter, N. (2019), “Challenges in implementing offshore wind power,” TLT, 75 (10), pp. 14-15.
- Shahriari, M., Cervone, G., Harding, L. and Monache, L. (2020), “Using the analog ensemble method as a proxy measurement for wind power predictability,” Renewable Energy, 146, pp. 789-801.