Today, wind energy contributes more than 6 percent of the nation’s electricity supply, more than 10 percent of total electricity generation in 14 states, and more than 30 percent in four of those states. However, as with other renewable energies, the wind-power industry still faces many obstacles. One of the biggest challenges to growth remains the high costs of constructing wind farms, as well as the ongoing operations and maintenance costs.
According to recent analysis [1] from New Energy Update, operations and maintenance (O&M) expenses cost between $42,000 and $48,000 per megawatt as an average during the first 10 years of a turbine’s operations, and wind-farm owners are expected to spend more than $40 billion on O&M over the next 10 years. As the need to lower O&M costs remains a persistent challenge in the wind-power industry, companies are beginning to explore the possibilities provided by emerging technologies such as artificial intelligence (AI) to boost performances, increase operating efficiencies, and achieve greater productivity. The recent advancement in these technologies, especially in AI, is digitally transforming the O&M world. AI-based blade inspection is a great example where emerging technologies such as computer vision, machine learning, drone automation, and big data analysis are combined in an innovative way to help automate the turbine inspection and deliver real-time data insight for wind farms to achieve greater profitability.
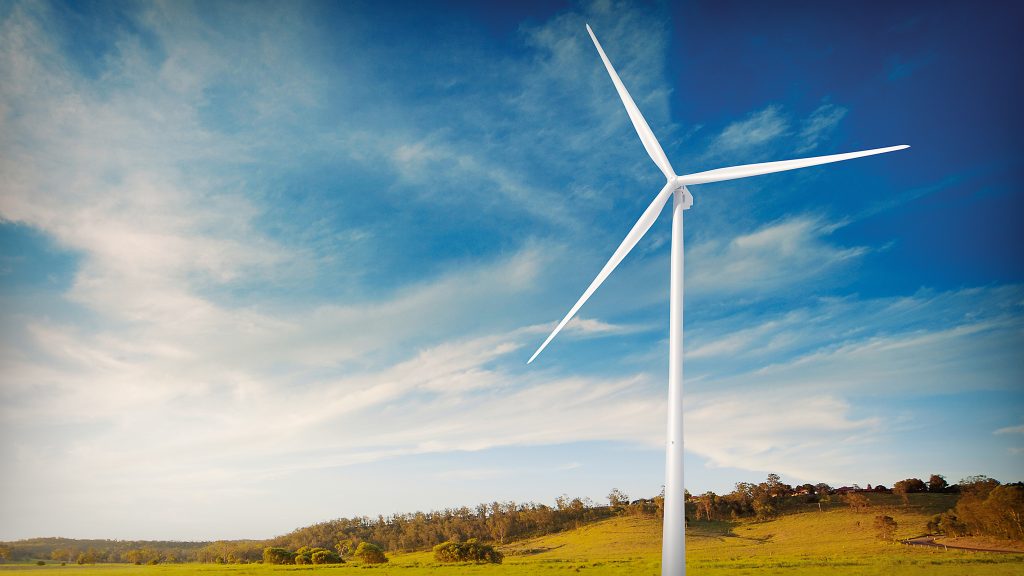
Challenging the Status Quo
Though many turbine blades today are equipped with a variety of IoT sensors measuring vibrations, sounds, and more, wind-farm operators still need greater — and earlier — visibility into the condition of blades. For instance, by the time a turbine has degraded to the point where it is vibrating or creating an unusual noise, the damage is already severe. Therefore, regular visual inspections of blades are necessary to identify cracks or other blade damage that can be fixed with a simple patch while still small.
However, traditional visual inspection approaches are labor intensive and require highly trained professionals. Traditionally, visual inspections could be carried out by either sending technicians up the tower on ropes to inspect the blades or by using ground-based scopes and cameras to capture defect photos from the base of a turbine. Wind assets have to be powered down for prolonged amounts of time, up to six to eight hours, for these types of inspections to be completed. In addition to the prolonged downtime, which otherwise could be used to produce energy, images captured may not be as complete and clear as needed. Due to the height of the turbine and the shape of the blades, the angle looking up the blades is very large, and some parts of the blades are in shadows while the other parts are under bright light. This naturally makes it difficult for ground-based scopes and cameras to identify all the defects and capture high-resolution photos of them. Small defects might be overlooked, and the picture quality might not be high enough for further severity diagnosis and damage analysis.
In the past few years, drone inspections have gained a lot of attention. However, manually-operated drones have limitations, too. The effectiveness of drone inspections depends highly on the drone operator. During an inspection, the operator needs to know exactly when and how to adjust the drone to accommodate the current wind speed and direction, so the drone doesn’t accidentally crash into blades and cause severe damage. Therefore, the operator needs to be a highly trained professional. This dependency on highly-trained drone operators prevents wind farms from using manual drone inspection as frequently as they would like.
Fortunately, these are about to change by using fully autonomous drone inspections, thanks to recent advancements in artificial intelligence, especially computer vision (CV) and machine learning (ML), which made reliable autonomous inspections possible.
Automating Drone Visual Inspections with AI
There are many forms of artificial intelligence, all of which emphasize the creation of intelligent machines that work and react like real humans. Computer vision is a technology that trains the machines to acquire, analyze, and categorize visual images or videos, mimicking the tasks that human visual systems do. Algorithms are the core of a computer vision system, and it is essential to a fully autonomous visual inspection.
What constitutes a fully autonomous inspection? Similar to the current market offerings of self-driving cars, there are various levels of autonomy for drone inspections in the market. For a fully autonomous drone inspection in the market today, it means a drone should take off on its own, locate the turbine blades, complete the inspections, and land back at the starting point.
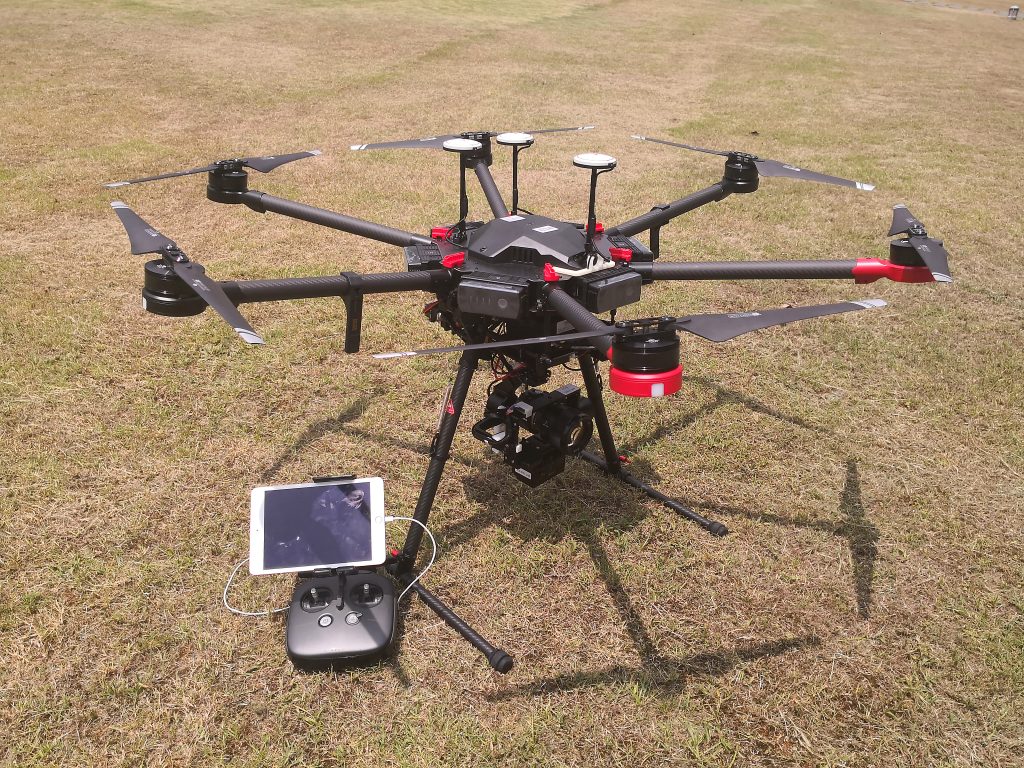
How does computer vision help? When the drone is in the air, its onboard computer-vision algorithms help to identify the blades and determine the flight path. An optimized solution should not be a pre-programmed route, so it doesn’t limit itself to any specific make or model of turbines, turbine heights, blade-stopping positions, or location. The advanced computer vision algorithms onboard give “eyes” and “brains” to drones, so they can make the most optimal flight path decisions in the air, precisely track the blades during the entire inspection, and completely cover all the surface of every blade. Therefore, there is no longer a dependency on highly trained human operators for visual inspections, and their expertise could be saved for more high value tasks.
In addition to take-off, landing, and path finding, autonomous drones have another critical mission during each inspection: to capture high quality blade images and data. For a drone to complete an entire turbine inspection within 15 minutes, all pictures need to be captured while the drone is in motion — with no time to stop. This creates additional challenges for image clarity. Fortunately, advanced algorithms are used to automatically adjust the camera’s settings — including focus, exposure, and white balance — so that image quality can be consistently high even when the drone is flying or when blades are under poor lighting conditions. Currently, the leading autonomous inspection system shows promising results, in that it is able to automatically identify and flag defects such as hairline cracks or chips as small as 1 mm by 3 mm. In fact, autonomous drones are so precise that they have even been known to capture and identify a common fly resting on a blade during inspection.
By reducing the inspection process from days to minutes with improved accuracy, wind farms no longer need to have their turbines powered-down and not generating electricity for prolonged periods of time. They’re also able to dramatically reduce the amount of labor involved, both of which help the farms reap tremendous cost savings. Using autonomous drones for visual inspections has demonstrated enough promise such that analysts estimate [2] the market to grow at a compound annual growth rate (CAGR) of 12.93 percent during the period of 2017 to 2021 as more and more farms start adopting this technology.
Better Predictive Maintenance with AI
Automated data collection is only the beginning of the benefits. By leveraging computer vision and machine learning, such autonomous drone solutions can analyze huge volumes of data in the cloud, almost instantaneously, and deliver real-time insights that can help wind farms identify trends and make decisions to improve operational performance. Recent advances in edge computing are enabling this type of real-time data analysis. Because wind farms are often in extremely remote locations, transferring data from turbine sensors to a centralized location not only takes time, but it is also limited by lack of bandwidth to these areas. With real-time data analysis at the edge, wind-farm operators can make critical decisions faster, such as shutting down a turbine to avoid cascading damage. Moreover, with cloud-based reporting, they can easily track the entire lifecycle of turbines, see the progression of damage, and view trend reports on individual turbines or their entire fleet.
In addition to using computer vision and machine learning to identify necessary repairs and existing blade damage on turbines, some wind farms are beginning to go a step further and use these technologies to predict when faults will occur and schedule maintenance before it’s needed. As mentioned earlier, the longer a wind farm waits to fix a problem, the costlier it becomes since powered-down turbines are not producing electricity. With the real-time data from visual inspections and turbine sensors, wind-farm operators can understand the growth rate of defects and determine if component failure is imminent, or if repairs can wait for a more opportune time. Predictive and preventative maintenance allows operators to save time and money by scheduling maintenance in advance, avoiding long downtimes, and scheduling repairs for times of year when weather conditions are best.
References
[1] newenergyupdate.com/wind-energy-update/us-wind-om-costs-estimated-48000mw-falling-costs-create-new-industrial-uses-iea
[2] www.researchandmarkets.com/reports/4225871/global-wind-turbine-inspection-drones-market-2017