The energy transition from fossil fuels to renewables is fully underway. Concerted action by industry leaders and investors is set to rapidly accelerate renewable energy growth. Although momentum is gaining, new approaches to asset optimization are needed to make best use of the influx of capital into projects.
DNV’s “Energy Transition Outlook 2021” forecasts that wind capacity will reach 2 TW by 2029 and 5.9 TW by 2050, contributing to more than 30 percent of the global-energy supply. However, the levelized cost of energy (LCOE) from wind will need to be reduced by more than 40 percent to enable this.
To date, much of the decrease in LCOE for wind farms has been driven by economies of scale in the supply chain and increases in turbine size.
But wind-asset owners and operators looking to boost the productivity of their existing portfolios will need new strategies to ensure they are taking advantage of all available opportunities to improve the financials of their investments.
The latest advances in wind-farm optimization technology make one of the “holy grails” of wind asset management possible — automatically boosting production across an entire site, achieved by focusing on the performance of the entire farm instead of individual turbines.
Made possible by the Industrial Internet of Things (IIOT), this new approach to wind-farm optimization, called swarming, will tackle the root causes of underperformance and unlock billions of dollars in extra revenue for wind investors globally. This article will explain how swarming can provide wind-farm operators with a 3-5 percent improvement in annual energy production.
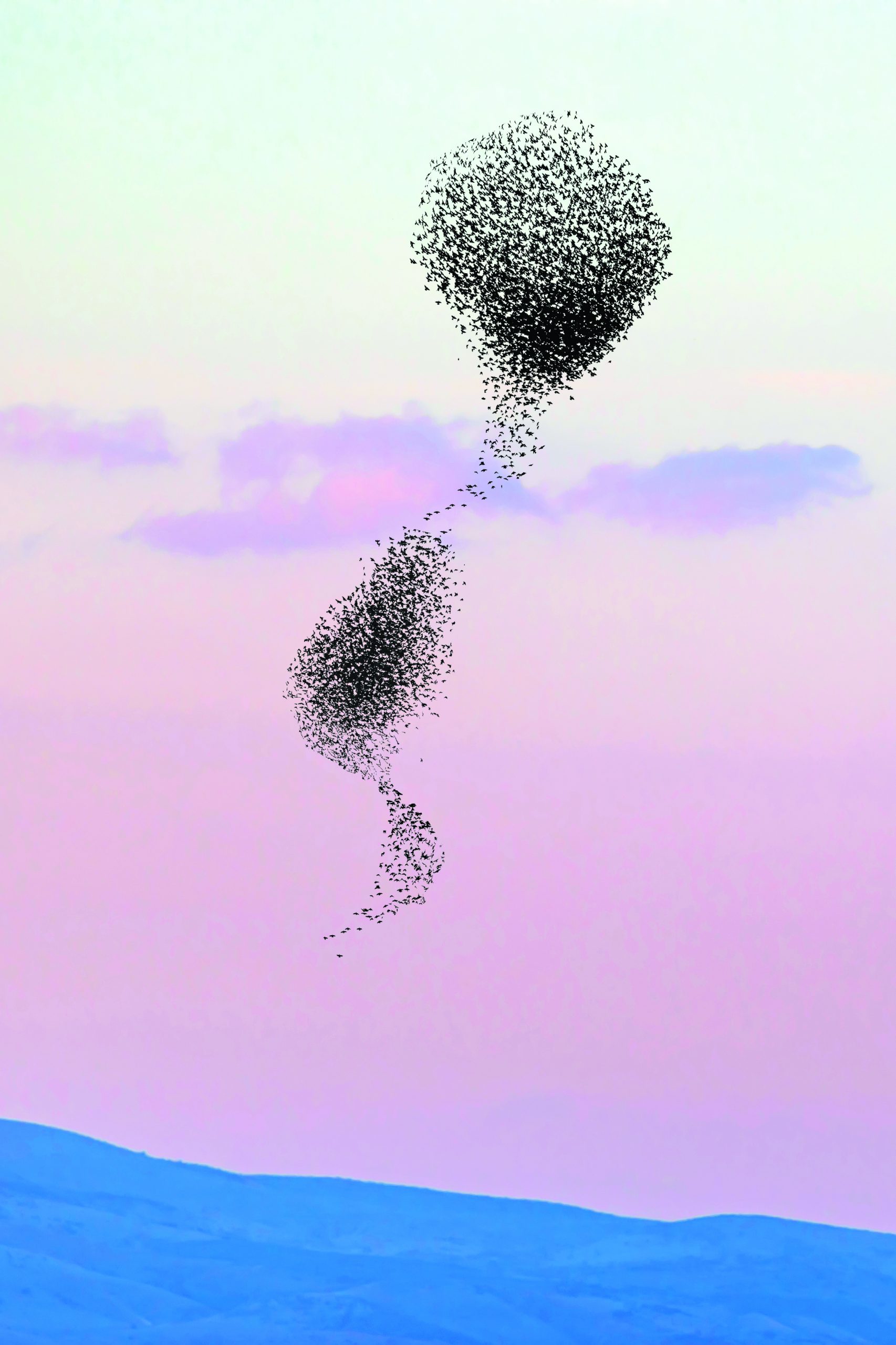
The Underperformance Challenge
The causes of wind-farm underperformance are complex. Even as power prices trend down in the long-term, reducing profitability, costs have started to increase. Larger rotors unlocking higher capacity factors have helped offset this decline in power prices but have, in turn, increased repair costs for blades.
The increasing scale of wind turbines has also driven wider manufacturing and maintenance costs up, which has further been exacerbated by supply chain issues, a shortage of skilled labor, and higher commodity prices.
Ironically, despite wind energy playing a key role in moving to a low carbon future, climate change is also affecting the industry. Extreme weather events are on the rise, leading to damaged wind plants and higher insurance costs. Once consistent weather patterns are also becoming harder to predict globally. Ensuring asset reliability in an uncertain world is vital.
The upshot is that widespread underperformance of wind farms, if not tackled swiftly, could leave the wind sector with one arm tied behind its back as it navigates a series of pivotal challenges.
Wake Effects
One common cause of energy loss is wind-plant wakes. A myriad of studies show that between 5 and 20 percent of a wind plant’s output is lost due to adverse turbine-to-turbine interaction and the wake effect, where upstream turbine operation negatively affects downstream turbine performance — and the collective of the performance of the wind plant as a whole.
Wind-plant size and internal spacing are important factors in determining the extent of wake effects. In general, the larger the wind plant and the more tightly packed turbines are within the plant, the larger the wake losses. Significant wake losses are often experienced by offshore wind farms or within large plants installed in markets with high power prices and limited land availability.
As rotor sizes continue to increase and the optimal onshore wind sites fill up, newer projects will become more exposed to wake-related performance risks. Feeding off this trend, repowering projects to retrofit larger rotors onto tightly spaced older and smaller turbines also lead to enhanced wake losses.
Ultimately, these factors point to the need to rethink the way wind plants operate. We are living in an increasingly interconnected world. Individuals, businesses, and governments are taking the value of cooperation on board. Driven by and enabling this, new digital technology is being used to interconnect everything from household appliances, to automotives, to healthcare devices.
Despite this, wind turbines continue to operate in isolation. Each wind turbine is treated as a standalone problem to solve.
Whether a wind-farm operator is managing a 10- or 100-turbine wind plant, operators will essentially use the same control strategy that focuses on maximizing the output of each individual turbine, so that each turbine boosts its output based on its own sensors and current inflow conditions.
No information is exchanged between turbines, and the innate interconnectedness of the site is ignored.
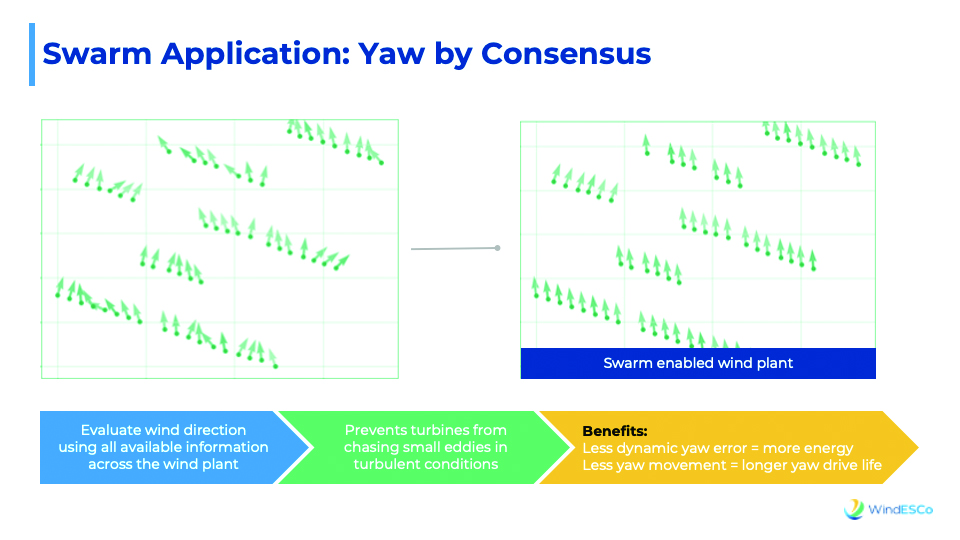
Inspired by Nature: Swarming Wind Turbines
The key to unlocking the potential of existing and new wind assets is to connect the dots and ensure wind turbines are sharing and acting on crucial data streams to operate for the benefit of the entire farm, boosting site-wide production instead of individual units in the farm.
The inspiration for this approach, and the technology which underpins it, comes from nature — more specifically, the concept of a swarm.
Swarming in Nature
In nature, swarming is the collective motion of a large number of insects, birds, fish, or tetrapods (four legged animals). Due to the complexity of these formations and the benefits conferred to the animals, this has been a strong area of research for artificial intelligence.
Ants, for example, are simple and not particularly effective when acting alone, but as a colony, they perform complex, coordinated activities far beyond the awareness of any individual ant.
Another famous example is starlings, which perform dazzling “murmurations” at specific times, which can involve tens or even hundreds of thousands of birds. They fly in complex formations, without collisions, by communicating with and maintaining an awareness of just a few of their neighbors. In the case of migratory birds flying in a V formation, the cooperation enables the birds to reduce their energy expenditure between 12 to 20 percent while flying long distances.
Swarming Technology in Wind
Swarming combines hardware and software as an integrated system to help owners unlock value by allowing turbines to communicate with and learn from each other.
WindESCo used a multidisciplinary approach to develop the system, combining the fields of turbine loads, controls, meteorology, sensing, and machine learning. The result is a 3 to 5 percent improvement in annual energy production (AEP) by continuously and autonomously optimizing the site as a single system.
The retrofit Swarm application can be divided into four layers:
Swarm Software/Applications: The core software layer running on the Swarm Server that communicates with all the Swarm Edge devices and makes real time optimization decisions.
Swarm Edge: The IIoT edge device and software connected to the turbine controller gets real time turbine-level data and implements cooperative control.
Swarm Server: The wind plant level server within the firewall that communicates with each Swarm Edge and the wind plant SCADA server.
Swarm Cloud: The offline data analytics and model optimization layer that provides longer term analytics and visualization of Swarm effectiveness at a wind plant.

How does WindESCo Swarm Work?
WindESCO Swarm offers multiple value propositions through separate applications, which together increase AEP between 3 and 5 percent at the wind-plant level. Several of these applications implemented as part of the Swarm system are briefly defined as:
1. Yaw by consensus
When each turbine operates independently, it must rely solely on its sensors to determine the wind direction and appropriately modify its control settings. These sensors are typically mounted on the turbine nacelle and measure the flow direction at a signal point near the center of the rotor, which doesn’t adequately characterize the average flow through the wind-turbine rotor sweep.
By combining measurements from varying subsets of turbines in the wind plant, the wind-plant complex flow field can be comprehensively characterized, and the yaw positioning of individual turbines can be optimized to ensure maximum energy capture. This sharing of wind knowledge means all turbines can improve their performance.
2. Wake Steering of the Site
Wind plants lose between 5 and 20 percent of their output to wakes. One of the most effective strategies for mitigating wakes to increase the overall plant production is wake steering. In wake steering, the yaw position of upwind turbines is modified so they no longer face directly into the wind. As a result, the downstream propagation of wind turbine wakes is deflected, or steered, away from the downstream turbines.
The neighboring downstream turbines are then exposed to undisturbed, higher velocity flow and are able to generate more energy. Although the upwind turbines will produce slightly less power than if they were pointing directly into the wind, the wind plant as a whole will generate additional power as a result of the wake losses being mitigated.
3. Predictive Yaw
Traditional yaw controllers are slow and rigid, and use wind-direction information at each turbine to make yaw control decisions. Yaw control strategies are defined by the OEM for a specific set of generalized site conditions; however, decisions about when to yaw are often suboptimal due to differences from the modeled site conditions.
Swarm-enabled predictive yaw uses optimal search method techniques combined with machine learning to identify how wind variabilities are propagating through the wind plant and to determine the wind plant global wind direction, thereby allowing turbines to make optimized yaw control decisions given the current site conditions. This system enables proactive yaw actions to increase energy capture and preemptive yaw actions to protect turbines from extreme events.
Swarming Case Study: Longroad Energy
Boston-based renewable energy developer, owner and operator Longroad Energy is a long-time customer and partner of WindESCo. The two companies have worked together on wind-asset optimization since 2018.
Longroad is one of the first businesses to optimize AEP across the entire fleet, rather than individual turbines, seizing the opportunity opened up by new AEP optimization technologies. WindESCo Swarm was recently deployed by Longroad Energy to optimize the 306-MW Milford Wind project in Beaver County, Utah, unlocking site-wide AEP gains of 3 to 5 percent for their 165 GE and Clipper turbines.
By adopting a proactive strategy for loss mitigation and revenue growth, Longroad is set to benefit from healthier, more productive assets while seeing a significant return on investment.
What’s Next for Wind?
Wind investors and asset managers have a broad suite of tools available to deliver the efficiency gains a smooth energy transition requires.
There are compelling industry-wide reasons for optimizing the global wind fleet. However, the basic choice available to operators is whether they can afford not to take advantage of technology that transforms revenue for far less than traditional repowering strategies. And as ever, the biggest winners will be those which move first.